Relationships – be they professional, romantic, platonic, or familial – govern and guide our interactions and wellbeing as humans: in our most exposed and naked form, we are social animals.
As public health scientists, we identify and research relationships, primarily between exposures and outcomes. But these terms are empty buckets that can be filled with anything from genetic markers (trisomy 21, BRACA2) to environmental conditions (pollutants, parasites) to medications (standard-of-care therapy, investigational drugs) to coaching (safe sex, parenting classes), and much more. What is deemed an exposure in one study—take hypertension, for example—could be an outcome in another. These terms are not static or inherent qualities, but rather indicators that orient and explain the nature of the situation under analysis. Unlike a biologist or historian, an epidemiologist is a methods expert rather than a content expert.
The beauty and complexity of relationships is that they rarely exist in a vacuum.
As scientists interested in causality, we need a way to focus on a singular relationship between two things—the exposure and outcome of interest—and remove all the noise. As public health researchers, however, we study real life and rarely have the luxury of creating artificial or laboratory environments like other fields in science. Confounding describes the ever-present noise, and controlling for confounding is the distillation process that allows us to hone in on the relationship of interest.
Defining Confounding
Confounding could be synonymous with distortion. The confounding variable distorts or changes the strength of association between the exposure and outcome of interest—in any direction and at any magnitude.
Scientific literature provides the following definitions:
- Confounding is said to be present when the relationship between an exposure and an outcome is strengthened, weakened, induced, or eliminated by a third variable or group of variables.1
- Using the counterfactual model, confounding is said to occur when the exposed and non-exposed groups are non-interchangeable.2
Diving deeper, there are three criteria of a confounding variable:
- It is causally associated with the outcome of interest.
- It is either causally or non-causally associated with the exposure of interest.
- It does not lay in the causal pathway between the exposure and the outcome. ((Szklo M, Nieto FJ. Epidemiology : beyond the basics. Fourth edition. ed. Burlington, Massachusetts: Jones & Bartlett Learning; 2019.))
How to Identify Confounders
There are three common ways to identify a confounder.
The first step in any research endeavor is to review the existing literature. Good research never takes place in isolation; it is based on the previous studies. For example, age and ethnicity are known confounders for many conditions, such as cancer and cystic fibrosis, respectively.
Second, visual models, like directed acyclic graphs (DAGS), exist to help illuminate the causal pathway and identify any other factors that may be affecting or influencing the relationship between the exposure and the outcome. ((Greenland S, Pearl J, Robins JM. Causal diagrams for epidemiologic research. Epidemiology. 1999:37-48.)) For common diseases like heart disease, visual models likely already exist in the literature. For less common or researched conditions or outcomes, you will likely have to construct your own models in collaboration with subject matter experts.
Third, there are analytical techniques that can demonstrate if confounding may be present. In each of these techniques, the aforementioned three criteria for confounding must be mathematically demonstrated.
- To demonstrate that a relationship between the outcome and potential confounder exists, the odds ratio (OR) and/or risk ratio (RR) should not equal 1. For categorical variables, the Chi-squared test should have a statistically significant p value.
- To demonstrate that a relationship between an exposure and potential confounder exists, the OR and/or RR should, once again, not equal 1. Again, for categorical variables, the Chi-squared test should have a statistically significant p value.
- To demonstrate that the confounding variable changes the magnitude of the relationship between the exposure and outcome of interest, the data should be stratified on the confounding variable. The magnitude of the relationship stratified on the possible confounding variable should be significantly different than the magnitude of the unstratified relationship. Bear in mind that while there are existing norms suggesting how to quantify a significant difference (e.g., a change in estimate greater than 10% or a p value of less than .05), as the researcher, you have the autonomy to articulate these criteria and operationalize this term before beginning the study. In fact, even when employing analytical techniques that do not mathematically indicate that a particular variable is a potential confounder, you, as the researcher, may still (wisely) choose to include it in your model based on the existing research.
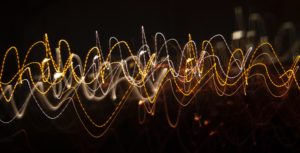
Controlling for Confounding
Once we recognize these potential confounders, these distorting factors, we have various options to remove their influence. This process has been codified in epidemiological jargon as adjusting or controlling for confounders. By distilling the relationship between the exposure and outcome of interest, it could be said that we are finding the signal in the noise. This can be done at two different points in your research: the design phase or the analysis phase of a study (or both!).
Design-Phase Techniques
In the design phase, there are three primary ways of controlling for confounding: 1) restriction, 2) matching, and 3) randomization. These techniques can be used individually or simultaneously, and each has its own advantages and disadvantages.
First, researchers may employ restrictive study criteria that prevent a person with a certain characteristic (the confounder) from entering a study. Restrictive study criteria could include a static or inherent demographic variable (e.g., sex or a specific age range), practicing a specific health behavior (e.g., smoking status), diagnosed underlying comorbidities (e.g., obesity or hypertension), or holding certain beliefs and attitudes (e.g., vaccines lead to autism). By restricting the study sample, the researcher removes the effect of that distorting force or confounder on understanding the relationship of interest (between the exposure and outcome of interest for the study). This approach is applicable to almost any study design. However, exercising this power comes at a cost to the research team, as restrictive criteria limit the external validity, or generalizability, of their study results.
Another approach is for researchers to match study participants in different arms of the study based on confounding factors. Matching is commonly conducted in case-control studies; however, it could also be used in other studies designs, like cohort studies. Matching is a bit more complex and can be accomplished in three ways: 1) individual matching, 2) caliper matching, and 3) frequency matching. Individual matching is the most intuitive: cases and controls are matched, or paired, based on shared characteristics. Finding an exact individual match can be challenging, so researchers may use caliper matching to match cases with controls that differ by only a pre-specified amount. Propensity score matching is effectively an aggregated caliper matching approach where all covariates are aggregated into a singular propensity score and then matched with controls who share a score with a pre-specified amount of difference. Finally, frequency matching emerged as a more feasible alternative for larger data sets. The matching no longer takes place on an individual level; instead, it happens proportionally, across the strata in the entire data set. Common examples of matching criteria include age, race, and location.
Randomization is another design-level technique to control for confounding: each participant has an equal chance of being placed in either or any of the study arms. This approach is not applicable to all study designs and is most well suited for randomized trials. If conducted correctly, letting chance determine each study participant’s placement creates comparable study groups with respect to both known and unknown confounders. Moreover, an additional benefit is that it can eliminate possible selection bias. Of note, this technique does not guarantee comparability; it simply and significantly reduces the potential for confounding variables.
Analysis-Phase Techniques
There are two common analysis-phase techniques that can be used to deal with confounders. As discussed above, stratification is an effective way to identify a confounder. The Cochran-Mantel-Haenszel method is a permutation of stratification that creates a single weighted effect estimate and accounts for confounding. While it is the simple choice that can be conducted using the famous epidemiological 2×2 table or statistical software code, it is also a limited option, as it can only adjust for one confounder and requires a categorical variable. This approach is often used in a preliminary analysis and is effective for looking at a detailed level of the data to elucidate associations in specific strata.3
Another analysis-phase technique that can be used to deal with confounders is multivariate regression modeling. In a regression model, the relationship between an exposure and outcome of interest can be assessed while adjusting or controlling for many possible confounders simultaneously. Moreover, this technique can accommodate and assess continuous confounders, or noncategorized covariates. While multivariate regression is more complicated than other techniques and typically dependent on statistical software, it is also a more robust and common practice, especially when looking at big data sets. Importantly, there are many different types of regression models, (e.g., linear, logistic, and Cox regression models), many different ways to build models (e.g., backwards, forwards, stepwise), and many different assumptions (e.g., normally distributed data, no collinearity, constant rate of change over time) that will influence the validity and interpretation of the results in a regression model. Therefore, such an approach must employ prudent, intentional, and a priori directives to eschew its misuse and incorrect interpretation.
Praxis
Putting the theoretical knowledge and techniques into use
Contextualized guidelines and best practices are more useful than Kantian absolutes when conducting public health research. Therefore, this article lays out the strengths and weakness of different techniques from both a theoretical and practical perspective.
In general, proactively addressing confounding in the design phase is better than reactively addressing confounding in the analysis phase. However, some techniques like randomization may not be applicable to every study design, and other techniques like matching may limit the external validity of the study. Physics has the Heisenberg uncertainty principle: you cannot ever know the exact speed and location of an atom. Here we introduce the validity uncertainty principle, which states that a study can never be perfectly internally and externally valid. There is always a tradeoff between internal validity (accuracy) and external validity (utility and generalizability) in a public health study.
Moreover, it is always possible that the aforementioned techniques will not fully control or adjust for the confounder, a phenomenon called residual confounding. In this case, some level of confounding is still present in the data.
Epidemiologists often intentionally employ categorical variables rather than continuous variables due to their ease of interpretation. However, a key assumption of using categorical variables is that the risk or effect of a confounder is the same within the entire category. If assessing age as a confounder, for example, the assumption would be that the effect of age is constant throughout the given range. If your age categories are 18-50 and 50 and older, you would assume that an 18-year-old and a 49-year-old carry the same risk, while a 50-year-old and a 95-year-old carry the same risk. Given differences in biology, behaviors, and the built environment, it is not uncommon for residual confounding to be present when age is categorized, especially with large ranges.
Equally important to thoughtfully articulating and developing an analysis plan a priori is the intentional and clear reporting of these methods in research papers. Even with published and available guidelines4 and resources,5 studies have shown that a large portion of research either did not report6 or conducted an incorrect analysis. Replicability is a key pillar of scientific research, and, as scientists, we need to both responsibly conduct and publish research and review the literature that is being published.
There is a multiplicity of ways to identify and control for confounding in any study (including less-common techniques not discussed in this primer), and the strategy identified and selected will depend on many factors, such as findings from the existing research in the field, the study design and data collection methodology, and practical considerations (e.g., time, budgets). Moreover, employing one or more techniques to adjust or control for confounding does not guarantee its removal. The validity uncertainty principle reaffirms that there is always a tradeoff between the accuracy and generalizability of our research. Yet both are critical to developing and disseminating equitable, sustainable health care solutions that achieve our shared epidemiological goal of studying the distribution and determinants of health-related states or events (including disease), and the application of this study to the control of diseases and other health problems.7 The techniques and tactics discussed here can be considered universal recommendations: articulate an a priori research plan that includes specific techniques and cutoff levels for significance, use a DAG, address confounding in the design phase when possible, and clearly report your methods in a manuscript so that other research teams can easily replicate your results.
Relationships guide and govern both our interactions as social animals and the associated data generated by these behaviors and outcomes. In turn, research evaluates our everyday activities and realities. Therefore, in our intertwined, interconnected world, effective researchers must be able to navigate through the noise to identify, isolate, and accurately measure relationships.
- A New Year, A New Needed Perspective - November 17, 2022
- Navigating the Line Between Discomfort and Uncomfort: Exploring Cultural Immersion - November 2, 2022
- Locating Eckhart Tolle’s “A New Earth” Within Current Public Health Epistemology: A Book Review - October 12, 2022
- Szklo M, Nieto FJ. Epidemiology : beyond the basics. Fourth edition. ed. Burlington, Massachusetts: Jones & Bartlett Learning; 2019. [↩]
- Rothman KJ, Greenland S, Lash TL. Modern epidemiology. 3rd ed. Philadelphia: Wolters Kluwer Health/Lippincott Williams & Wilkins; 2008. [↩]
- Kahlert J, Gribsholt SB, Gammelager H, Dekkers OM, Luta G. Control of confounding in the analysis phase – an overview for clinicians. Clin Epidemiol. 2017;9:195-204. [↩]
- Von Elm E, Altman DG, Egger M, Pocock SJ, Gøtzsche PC, Vandenbroucke JP. The Strengthening the Reporting of Observational Studies in Epidemiology (STROBE) statement: guidelines for reporting observational studies. Annals of internal medicine. 2007;147(8):573-577. [↩]
- Simera I, Moher D, Hirst A, Hoey J, Schulz KF, Altman DG. Transparent and accurate reporting increases reliability, utility, and impact of your research: reporting guidelines and the EQUATOR Network. BMC medicine. 2010;8(1):1-6. [↩]
- Walter S, Tiemeier H. Variable selection: current practice in epidemiological studies. European journal of epidemiology. 2009;24(12):733-736. [↩]
- Samet JM, Woodward A. On being an epidemiologist. American journal of epidemiology. 2019;188(5):818-824. [↩]